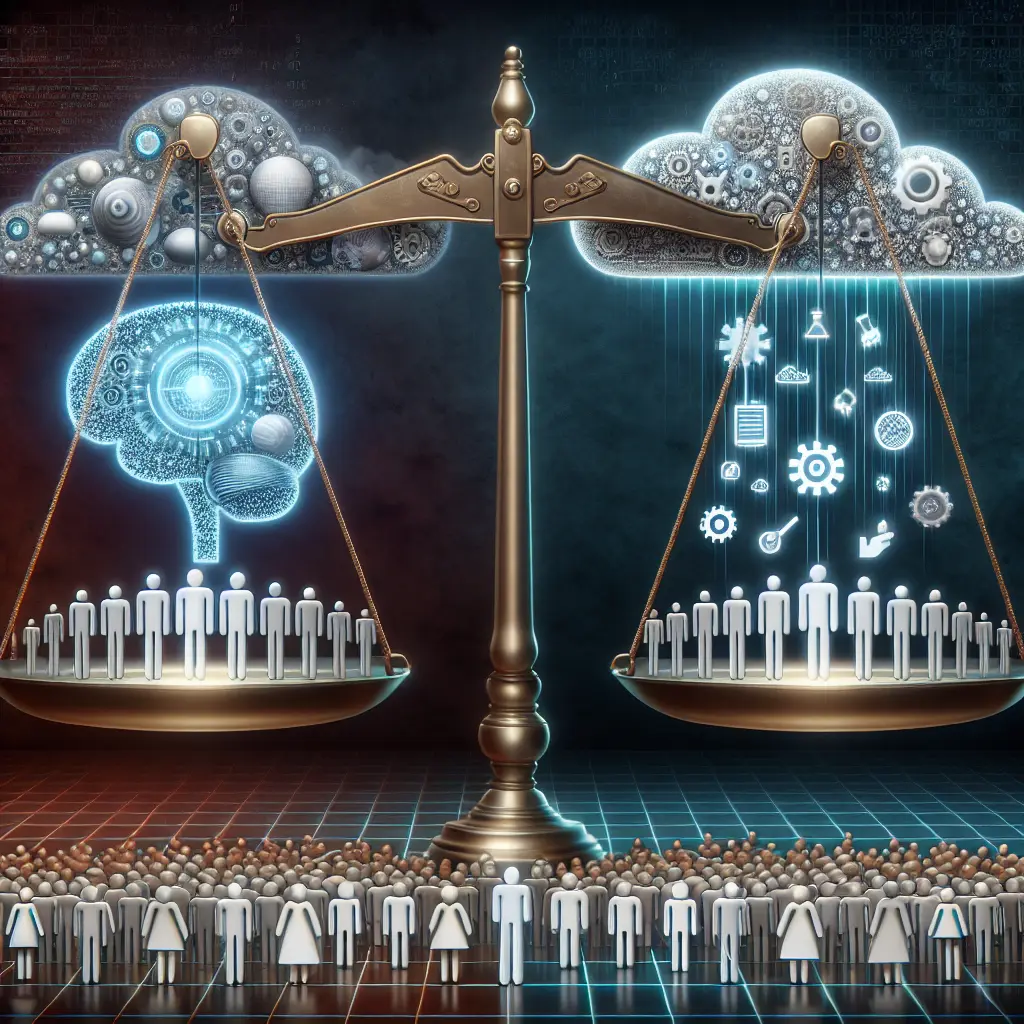
Exploring the Role of Bias Mitigation in AI Fairness
In today's rapidly evolving technological landscape, artificial intelligence (AI) has emerged as a transformative force across various industries. However, the increasing reliance on AI systems has brought significant concerns surrounding AI fairness and ethical AI practices to light. Central to these discussions is the pressing need to address bias in artificial intelligence, which can lead to unfair outcomes and perpetuate existing societal inequalities. Exploring effective bias mitigation techniques is crucial for reducing bias in AI and ensuring fairness in machine learning processes.
In an era where AI plays an integral role in shaping numerous sectors, the pursuit of AI fairness and ethical AI practices has become increasingly important. Addressing bias in artificial intelligence is crucial to ensuring fairness in machine learning processes and preventing AI discrimination. This section explores how bias mitigation techniques impact AI fairness by integrating recent developments and case studies to underscore their significance.
The Importance of Bias Mitigation Techniques
Bias mitigation techniques are essential for reducing bias in AI systems, often stemming from biased training data or algorithmic oversights. These techniques are vital for promoting equitable AI outcomes and fostering inclusive AI systems. According to a study published in the Journal of Artificial Intelligence Research, effectively implemented bias mitigation strategies can significantly reduce instances of algorithmic unfairness, thus upholding the principles of algorithmic fairness and responsible AI development.
One innovative application of AI is Joas Pambou's app, which integrates vision language models (VLMs) and text-to-speech (TTS) technologies to audibly describe images. This tool exemplifies how AI fairness strategies can enhance inclusivity, particularly for individuals with sight challenges. By ensuring that AI systems cater to diverse user needs, developers can mitigate bias and create more accessible technologies.
Recent Developments in Bias Mitigation
Recent news highlights various advancements in the realm of AI fairness and bias mitigation. Apple has been proactive in addressing concerns related to data ethics, asserting that its Apple Intelligence was not trained using stolen YouTube videos. Instead, the company has acknowledged using subtitles from YouTube for a separate project. This move underscores the importance of ethical practices in technology development and reinforces the need for transparency in data usage Apple's Ethical Approach.
Additionally, Google researchers have developed a new weather prediction system that combines AI with traditional physics. This model, called NeuralGCM, aims to "bridge a divide" between traditional meteorological methods and modern machine learning approaches. By integrating diverse methodologies, Google's initiative highlights the potential for AI bias solutions to enhance accuracy and fairness in scientific applications Google's Weather Prediction System.
Strategies for Reducing Bias in AI
To achieve fairness in AI, developers must adopt comprehensive strategies for mitigating AI bias. Here are some effective approaches:
Diverse Training Data: Ensuring that training datasets represent a broad spectrum of demographics is crucial for minimizing bias. Diverse data helps create more equitable AI models that reflect societal diversity.
Regular Audits and Testing: Continuous monitoring of AI systems can identify and rectify biases before they lead to unfair outcomes. Regular audits ensure that AI ethics are upheld throughout the development process.
Algorithmic Transparency: Making algorithms transparent and understandable can foster trust and accountability. Transparency allows stakeholders to scrutinize decision-making processes and advocate for necessary changes.
Collaborative Efforts: Engaging technologists, policymakers, and community stakeholders in discussions about fairness and bias in AI promotes shared responsibility and innovative solutions.
Case Studies Illustrating Bias Mitigation Impact
The integration of AI into design and creativity offers an intriguing case study on the impact of bias mitigation techniques. As explored in a recent article on the future of design, AI and machine learning are reshaping creative processes by offering new tools for designers. These technologies can foster inclusivity by providing creative opportunities to individuals from diverse backgrounds, thus reducing bias in creative fields Future of Design.
In the medical sector, AI's role in surgery illustrates how bias mitigation can lead to more equitable healthcare outcomes. AI-enhanced tools are augmenting human capabilities, improving surgical precision, and reducing disparities in patient care. By prioritizing fairness in machine learning applications, the healthcare industry can ensure that all patients receive high-quality care AI in Healthcare.
Challenges and Future Directions
Despite advancements in bias mitigation techniques, challenges persist. The dynamic nature of machine learning bias requires ongoing research and adaptation of strategies to stay ahead of potential biases. Furthermore, as highlighted by discussions on ethical technology practices, building trust in AI is imperative for its acceptance and success Ethical Technology Practices.
The future of AI fairness lies in cultivating responsible AI development that prioritizes ethical considerations alongside technological innovation. As we delve deeper into this multifaceted world, continuous engagement with ethical practices will be crucial for mitigating AI bias and advancing equitable AI solutions.
Engaging the Audience: The Path Forward
As we navigate the complex landscape of AI ethics and fairness, one question remains: How can each of us contribute to reducing bias in artificial intelligence? By staying informed about recent developments and actively participating in discussions on ethical AI practices, individuals can play a pivotal role in shaping a fairer technological future.
Join the conversation on responsible AI development and explore how we can collectively foster inclusive innovations that benefit society at large. Through informed discussions and collaborative efforts, we can ensure that the journey toward equitable AI continues to progress.
Conclusion: Navigating the Path to Equitable AI
The pursuit of AI fairness is a crucial journey that necessitates effective bias mitigation techniques to ensure ethical outcomes. The integration of diverse training data, regular audits, and algorithmic transparency are key strategies for reducing bias in AI systems. These approaches, coupled with collaborative efforts among technologists, policymakers, and communities, pave the way for responsible AI development. Notable advancements by tech giants like Apple and Google exemplify how ethical practices and innovative methodologies can address bias, enhancing accuracy and fairness across various applications.
Case studies from the creative and medical sectors underscore the transformative impact of bias mitigation. AI's role in these fields showcases how inclusivity and equitable outcomes can be achieved, highlighting the potential for AI to be a catalyst for positive societal change. Nevertheless, challenges remain, requiring ongoing research and adaptation to anticipate and address emerging biases.
As we continue this journey, it's vital for all stakeholders to engage actively in discussions on AI ethics. By staying informed and advocating for responsible AI practices, we can collectively foster inclusive innovations that benefit society. We encourage you to share your thoughts and experiences on AI fairness and bias mitigation in the comments below. How can we each contribute to shaping a fairer technological future?
Together, we can advance toward an equitable AI landscape, ensuring that these technologies serve as tools for progress and inclusivity. Let’s embrace this path forward with commitment and collaboration.
Warm regards,
Harper Dawson